Why your research insights aren't making an impact
3 big mistakes and how to avoid them
RESEARCH
12/12/202310 min read
If you conduct research in a corporate setting (e.g., user experience research, market research, data science, etc.), your success is measured by your ability to deliver valuable insights that drive business decisions. It is not an easy job!
Picture this: You’ve spent months conducting discovery research to learn more about a critical issue for your company. You learn some exciting things about your customers, and you’re confident that this information will enable your product teams to design great solutions that outperform the competition. You tell your colleagues about it all, and they are eager to learn more!
This happens more often than you might think, and sometimes it’s a matter of how well you defined the questions in your research. However, research skill aside, there are a few mistakes that almost every researcher makes in their career that lead to “insights” going nowhere. I’ll go over three of the big ones and how to avoid them.
Hold on… what is an insight?
Let’s get something straight. “Insight” has become a buzzword. It’s used so often in different contexts that it’s basically lost its meaning: everyone that uses the word means something slightly different, which will become important later.
In any case, the original definition of the word isn’t super helpful:
Collins: “an accurate and deep understanding of a complex situation or problem”
Merriam-Webster: “the act or result of apprehending the inner nature of things or of seeing intuitively”
Cambridge: “a clear, deep, and sometimes sudden understanding of a complicated problem or situation”
These definitions of “insight” only tell us the outcome of having one: that you suddenly understand something very deeply. How do you produce an insight for other people?
Knowing what an insight is does not mean you know how to induce one in others
Some people have tried to create a formula for writing insights, like “context + behavior/mindset + why + next steps.” Others have come up with “you’ll know you have an insight when…” lists, where there are different criteria for insights to meet in order to qualify.
These usually include some version of the following:
Grounded in data (you didn’t make it up)
Explains the “why” (what you told them makes sense to them)
Pithy (short and sweet, memorable, and catchy, so they’ll remember it and tell other people)
Actionable (they know what to do with what you told them)
Surprising (it’s new to them)
There are certainly more characteristics that you could list, but these are the ones I find the most helpful. Some of them point to the mistakes you can make in the research process. Let’s get into that now, shall we?
1. Working alone
This is a mistake that hits ex-academics harder than most, especially if they transitioned to a corporate job recently. The more academic training you have as a researcher, the more likely you are to be a lone wolf. You may have worked in a lab and had research assistants, but your research planning, analysis, and reporting were probably done mostly by you alone. You might have gotten feedback from a peer or superior, but it’s your work.
Remember I mentioned that insights mean something different to everyone? That means not only do different people in your audience expect different types of information, but they are also starting from completely different places when it comes to what they already think and know! No bit of information is an “insight” to every human being.
Let’s look at that last “you know you have an insight when” bullet: It must be surprising. Surprising is not an objective characteristic, it’s relative to the audience! In fact, most of the criteria point to insights as something that’s subjective to someone else.
What classifies something as an insight is subjective to each person you share information with.
As a researcher, no matter where you sit in the organization, you usually have people who have something riding on the outcome of your work. They are waiting on your insights to make a decision or validate a decision, and they are your primary audience. This is good for you because the list of people is much smaller than if you were writing an article for “everyone,” so it’s easier for you to tailor the findings to your audience.
The problem is, if you plan, execute, analyze, and write the report without involving other people in the process, you are very likely to get blank looks on the big day. As a researcher in an organization, you need to figure out what’s on your stakeholders’ minds before you even conduct the study. You need to involve them in your planning to make sure that the outcomes you expect to get are going to answer the questions they have. You should invite them in to see data coming in so they are invested in the process, and you need to figure out a way to get them involved or aware of the things that happen to the data from collection to reporting.
If you do this well your stakeholders will come out feeling ownership over the insights that developed, and they will champion them for you before your presentation is even over. The worst case scenario for this mistake is failing to identify who your stakeholders and audience are before beginning research.
2. Skimping on synthesis
Once you’ve finished collecting data for your study, it usually needs to go through two steps: analysis and synthesis. Analysis is taking the noise and breaking it down into smaller, cleaner parts. Synthesis is putting the parts together into a bigger, meaningful picture. Analysis can take a while depending on how complicated your data is. Synthesis usually takes a lot longer, and in a fast-paced environment where a deadline is looming, it is the most likely part of the process to be sped up or skipped entirely.
What happens if you stop there? If the patient is waiting for the results, they are not expecting your entire analysis document, because most of it will mean nothing to them. You’ve presented findings, but not insights. Who cares if their heart rate variability was 150? But if you take that data point, compare it to the patient’s medical history and context for getting tested in the first place, and you realize that value is 3x the typical value for someone in their situation, you might be able to tell them what is wrong and what to do to improve. That last activity is the synthesis process which leads to “insights,” though a doctor might call it a diagnosis.
I hope you noticed that even though synthesis produced the insight, it couldn’t have happened without doing the analysis. Analysis needs to happen first, but then you need to shift gears and do something with that output to get something useful.
You can’t get insights from raw data, and if you stop after analysis for the sake of speed, you will get results, but not very useful ones.
In corporate research, a lot of researchers you ask will tell you they don’t get to spend as much time on synthesis as they would like to. This is to everybody’s detriment: research consumers don’t get as useful of an output from the study, and research practitioners look less competent than they are.
Avoiding this is a matter of project management: expectations need to be set at the beginning of any effort about what research is happening, what is expected out of it, and on what timeline. If you are looking for deep insights, you can’t rush the schedule; researchers can and will speed things up if needed, but the depth of understanding will suffer.
Not every study needs long synthesis times; a summative usability study on a simple landing page should be very straightforward. But if you’re trying to understand the needs of a new customer segment, you need to build in the time for synthesis.
3. Presenting facts without implications
If you recall, one of the requirements of a good insight is that it’s “actionable.” You may ask, respectfully, what the hell does that mean? This is another buzzword, which roughly means that the person on the receiving end of the information does not feel the urge to ask things like “so what?” or “what do you want me to do with that information?” Again, very subjective.
Your job is to guide your audience in terms of not just what to think, but how to think differently. Throwing data points at a group of people, as smart as they may be, will lead to as many conclusions as there are people in the room. Not only is it irresponsible to assume that everyone knows exactly how to use your research findings, but it’s also a burden you are placing on them.
People have shit to do. They’re looking for you to help make a decision, not to give them a puzzle to solve.
This is a tricky area for researchers because getting to an “actionable” insight blurs the line between learning and problem-solving. It requires you to take a step beyond the immediate data and make an inference, or a suggestion. In the world of user research, this can be several things depending on what you set out to do.
Now you may protest, you’re a researcher, not a designer. Not a developer or a strategist, solutions are not your job! Not to worry, making insights actionable does not necessarily mean telling people the answer.
Realistic examples
Here are a few examples of what an actionable insight could mean in different situations, starting with the simplest and going to the more gnarly problems.
Situation: You are doing a usability test and learn that people can’t read the text on a button because the text color is similar to the button color
Insight: In this case, you can directly suggest changing the button and/or the text color to ensure a contrast ratio of at least 4.5:1 (an industry-accepted accessibility standard). You don’t need to tell them which colors to go with; that’s not something your data can speak to, nor is it your job.
Situation: You are doing a cognitive walkthrough for a site signup experience and find that after people tried to open an account, they neglected to read both the terms and conditions and the very critical instructions on the following page, which they needed to complete the next step.
Insight: Here, there is more ambiguity about what the solution is. You can point to the problem that users are ignoring critical information, and you could potentially suggest something as vague as figuring out a better way to draw attention to the information, or as specific as presenting the information in a different part of the user journey where it is more immediately relevant. Your relationship with your partners and knowledge of how they like to operate is going to be helpful here to figure out the best way to frame your findings.
Situation: You are analyzing some web activity on your site and noticed that following the most recent update, the percentage of users that successfully logged in dropped by 30%, while the number of unique attempts had nearly doubled from the previous month.
Insight: Just based on this information, it may be hard to identify the root cause of the problem, because quantitative data doesn’t tell you why it’s showing those numbers. You should escalate this issue, but you might recommend getting some qualitative data on what’s happening to see whether it’s a technical problem, a usability concern, a massive influx of new/different users, or a combination of those. Sometimes, the course of action is to do more research!
Situation: You have done some contextual research on how customers decide on which products to buy and discover that customers get turned off by overly sales-y language, especially when they aren’t even sure the product is good.
Insight: You might come up with the insight “Aggressive sales pitches turn away potential customers at the signup step. Our current checkout page prioritizes calls to action and does not include details on the product details or value. Sales pages should instead focus on clarifying and summarizing the value proposition.” Note the formula here: it tells you the customer behavior, the reason behind it, and provides a direction for change based on what the current experience looks like.
Situation: You work for an e-commerce company that’s planning on expanding its offerings and have done some generative research trying to understand customer needs around shopping as a whole. You find that customers are spending more and more time shopping around and hate it because it takes them too long to find something to buy. They are then dissatisfied because they worry that they might have missed out on something better, while also feeling that they wasted enough time already.
Insight: Your insights here might be complex, bringing in existing psychology research about decision-making, especially how having too many choices actually make it harder for people to make a decision and feel good about it. You could point out that your company’s current metrics for success include time spent on the site, and how that’s misleading because in this case more time can mean less satisfaction. And you could point to the current strategy of bringing in more products as potentially creating more pain for your customers, and identify an unsolved problem in figuring out how to help customers make choices more efficiently and with greater confidence. Perhaps you could even raise the provocation “Is it better to have more products to choose from or fewer products of greater quality?” These types of insights provide frameworks for other people to leverage their expertise to solve the problem.
Takeaways
There is SO MUCH you can do with deep customer insights. With the right information and storytelling, you can influence (and improve) the overall user experience in a human-centered way that also benefits the company’s bottom line. But keep in mind the requirements for good insights! Yes, they have to be informed by good data, synthesized thoroughly, and worded compellingly. But if the people you want to influence weren’t aware and involved in the process in some way, your impact will always be limited.
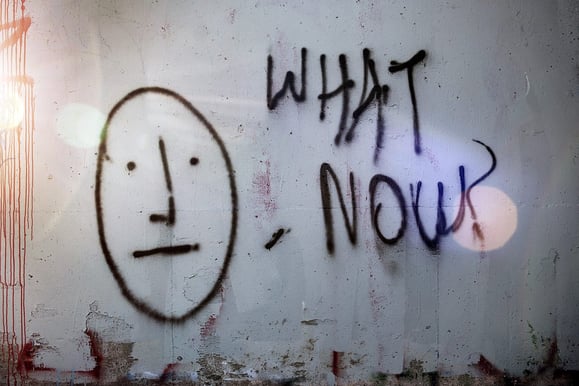
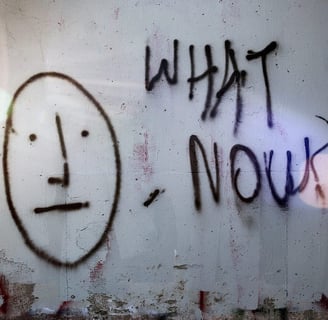
The big day arrives, and you deliver your presentation flawlessly to the sound of… crickets. Or worse, “Can you tell me what the insight is?”
Imagine you are looking at data that comes out of a heart rate monitor for a patient. It’s a bunch of electrical measurements that are useless in their raw form. Your analysis might include extracting the raw values from the machine and formatting it in a readable way, cleaning the data to remove the noise, capturing the patterns that indicate heartbeats, calculating the frequency, maximum and minimum amplitudes… all sorts of useful metrics.